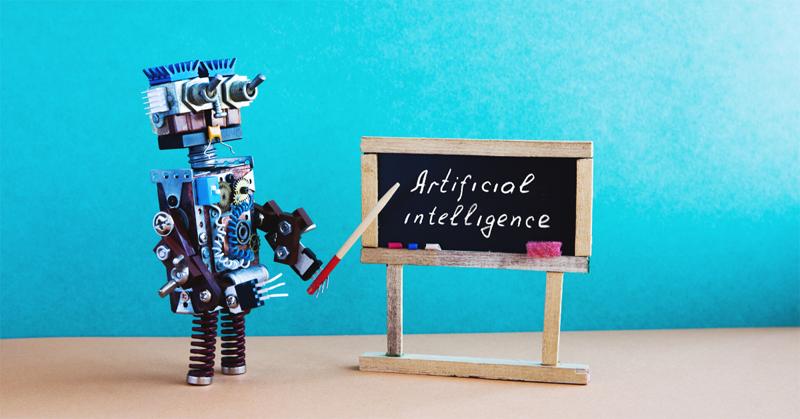
Abhishek Ray, Founder & CEO, Finarb discusses the importance of understanding business needs, accuracy, data quality and explainability when creating and implementing AI/ML solutions.
Tell us about you and your inspiration for setting up Finarb.
While working in consulting companies, as well as in the financial sector, I realised that organisations were not really using the full extent of their data and that was limited not only by an aversion to change but also with the fact that mostly people were not really aware of how data could be leveraged using the latest developments in the AI-ML arena.
That was what triggered me to try to bridge this gap here at Finarb. We not only focus on application of state-of-the-art algorithms in business to improve accuracy but also on explainability of models, automation of solutions, and fault tolerance. The goal is to provide clients with the 360-degree view of how AI/ML can improve their business.
How would you describe the potential of AI and Machine learning to improve the way the financial services firms operate?
There is a huge scope of application of AI and ML in financial services. In some of the use cases like credit risk, investment management, trading risk etc. we are already using the same to a large extent. However, there are several use cases where we see an opportunity to expand the use significantly. For example, in compliance, operation risk, personal financial management and recommendations.
If we take the use case of compliance, we have huge scope in ensuring regulatory requirements against information misuse, smoother operations and higher customer satisfaction. This involves speech to text models, advanced NLP models with low latency. This needs to be deployed in a centralised manner to transfer the learning from one customer to other customers. This involves not only a solution development overhead but also a technology shift in terms of devices, etc. This can act as a big deterrent for larger organisations but, in my opinion, the benefits far outweigh the costs and we are shifting – slowly but surely.
Where are we in the journey to creating computers that can think for themselves?
We are quite far from the position where machines can truly think or be conscious. Given the latest developments in AI, in certain use cases machines can emulate the way humans think. If we think about the multi-arm-bandit problem, there is no way where we can know the actual target distribution but through trial and error we can try to model the distribution based on the reward/punishment that is received at every trial and error.
Do you think that we have the right regulatory frameworks in place to deal with decision-making AI? Should we be concerned?
This is highly dependent on the industry. Also, this can be very easy to overlook in certain cases. The use of AI in defence or banking, for example, needs to be highly regulated to prevent mishaps or monetary losses. At the same time, say a bank emphasises equality in terms of gender and/or race. In that case, using gender or race in their models might lead to giving a preference to one gender over the other. Now this relation may be statistically significant or not, however the result is based on the data sample used by this bank. This result will unfairly penalise customers based on gender or race. The correct step is to remove such variables from the model and rebuild, which is not always done.
We spoke with your colleague recently about the strategic approach that companies need to take when implementing AI/ML solutions in their business. How do you bring your clients on the technology journey? Do they need to be technologists to understand the solution?
The first step is always to understand the client’s business. Every client is different in the sense, that they have different businesses, operate in different markets, have varying technology awareness, etc. But what is generally common is that they have a very good understanding about the nuances of the business. Hence when we would transition a client into the digital journey, we would talk not in terms of fancy tech or ML terms but rather try to impress the effects this transition would have on the business and how that can be possible. They do not need to be tech savvy to understand the needs and the effects of the solution.
The other important aspect is to show the incremental benefits to the client right from the very start of the project or as soon as possible. This way the customer has more confidence in the solution and it becomes easier to make the client a part of technology transformation journey.
What are some of the products that Finarb offers to clients that sets it apart from other companies?
We have two business verticals:
- Client based consulting and solutioning
- Product development
In either case we try to differentiate ourselves in a number of aspects. We put a big emphasis on data quality issues, smooth data flow, feature engineering etc and base the models used on the specific distributions that make sense for the business use case at hand.
We also focus a lot on automation of the end to end solution to allow the ML/AI to adapt to the changing nature of the business and its stakeholders. Lastly, we also focus heavily on the explainability of the model and continuous learning and allow the client to understand the nuances of the model and take part in improving the model with time through feedback.